Contents
2021, No 5
https://doi.org/10.11610/cybsec05, Full text (pdf)
- Aims and Scope
- Focus on the Issue:
- Latest in Cybersecurity
- Cybersecurity Institutions & Initiatives of the Issue: ESI CEE, CyResLab of ESI CEE, GCHQ and the Cybersecurity Lab at Sofia Tech Park
- Cybersecurity Institutions in Bulgaria and the European Union
Aims and Scope
Achieving a machine-based imitation of human cognition could have sounded like a futuristic dream a few decades ago, while nowadays, we are discussing its potential impact on human freedom, privacy, and life as we know it. Although still relatively far from answering Alan Turing’s question in 1950 – “Can machines think?”, the field of Artificial Intelligence (AI) has already reached a level of maturity, which allows people from different backgrounds to use its tools, such as Machine Learning (ML), Natural Language Processing (NLP), Computer Vision, Autonomous and Expert Systems for a variety of use cases, including within the healthcare sector, where AI bears an enormous potential. This potential has been mobilized in practice with the fight against the, almost a year old already, COVID-19 pandemic.
Although often mentioned as somewhat of a buzzword, the topic of AI has been present in the media forefront, even more so than ever, since the onset of the SARS-CoV-2 virus pandemic. And not surprisingly so – within the growing body of research in the field of AI and its related models and technologies, serious concerns about data privacy and security are often raised.
Machine Learning instruments have been widely used to analyze existing data and produce predictions regarding the virus spread, recognize patterns to help with the diagnosis of new cases, and help explain treatment outcomes. Another direction of the AI-based solutions to support the fight against the SARS-CoV-2 virus is for the rapid funnelling of drug compounds, that could prove effective against the virus, as well as for the potential side-effects analysis and medication effects predictions.
Against the backdrop of these applications, however, more controversial uses of AI have recently been reported, especially in the field of facial recognition, quarantine, and social distance control. As AI models need to process huge amounts of data, including sensitive and personal information, to be trained and integrated within a particular application, this makes them, firstly, vulnerable to attacks from malicious actors, and secondly, in some cases, questionably unethical.
In this issue of the newsletter, we would like to analyze and discuss those uses of artificial intelligence for the fight against the COVID-19 pandemic, through the prism of cybersecurity and data privacy and put forth propositions for companies and SMEs with relation to the ethical and safe use of AI means, methods and tools. Furthermore, and to give more context, we will provide an overview of some recent developments and interesting applications of AI methods and tools, in terms of AI against COVID-19, AI for Cybersecurity, Cybersecurity for AI, and Misusing AI. Last, but not least, we will try to convince our readers that securing artificial intelligence, will require new ways of thinking about security and what it means in the context of digital dependency and information society.
Issue Editors: Dr George Sharkov and Christina Todorova
Focus on This Issue: AI against COVID-19, AI and Cybersecurity, Cybersecurity for AI, and Malicious use of AI
The “new normal” imposed by the COVID-19 pandemic during 2020, has accelerated tremendously the deployment of experimental technologies in the area of healthcare, as well as and facilitation and security for virtualized teaming and teleworking. Likewise, a major boost was given to the adoption of AI following the explosive growth of investments during the past decade.
At the same time, a lesser-known fact is that this is the third boom of AI observed after its naissance in 1956. Back then, at the Dartmouth Conference, a small group of visioners agreed with John McCarthy’s conjecture that “every aspect of learning or any other feature of intelligence can, in principle, be so precisely described that a machine can be made to simulate it”. Great names, such as Claude Shannon, Marvin Minsky, Norbert Wiener contributed to the first boom of AI at the age of formal logical reasoning and perception, with amazing and promising prototypes, which were then followed by the first “AI winter” in the 1970s. Eventually, in the mid-1980s with the second boom of AI, we got much closer to human-like decision-making, based on knowledge representation models and expert systems, with acknowledgeable success in experimental medical diagnostics, biochemistry (like MYCIN, DENDRAL). Bulgaria has also demonstrated promising results in the applied areas of AI, such as biophysics (PREFES, KREBS at the Institute of Biophysics). However, scientists warned that to become a valuable decision-making adviser in real life, the expert systems would need better “common sense” knowledge and reasoning in addition to their formalized experts’ knowledge, plus the ability to process large data sets and huge computational power. Those were also the main reasons for the second “AI winter” in the 1990s.
Fast forward to our current age, for the third time, AI is yet again being pointed at as the “next big thing”. Yet now, this claim seems to be standing on much stronger grounds. The progress made in terms of computation power and methods, big data and analytics, computer vision and natural language, combined with the tremendous technological progress overall, made it possible to construct a collective artificial “brain” capable of winning against Gary Kasparov (1997, Deep Blue), win at Jeopardy (IBM’s Watson, 2011), beat world champions at Go by using Deep Learning techniques (AlphaGo, 2016), among many other achievements. This fueled new hopes for the future of AI and remarkably increased investments in AI. In 2018, the European Commission announced an increase of AI research and development to reach 1.5 billion EUR by 2020, catching up with Asia and the US. Shortly after that, at the beginning of 2021, the European Strategy on Artificial Intelligence has set a target to increase AI investment (public and private sectors combined) to at least EUR 20 billion per year, over the following decade. Meanwhile, in the USA, investments in AI companies increased from 300 million in 2011 to around 16.5 billion US dollars in 2019, and above 25 billion in 2020. Not surprisingly, China is already declaring a global leadership in AI research and proclaimed its ambitions to become the AI superpower of the world with 150 billion US dollars by 2030. And, in 2020, Bulgaria accepted a concept for the development of Artificial Intelligence in Bulgaria until 2030, focusing on scientific advancements and software/IT development, intelligent agriculture and healthcare.
Notwithstanding, the lockdown also gave a boost to the actual digital transformation of business, public administration, education, and social life and has forcedly activated plans belated for years. AI developments, although mostly in an experimental phase, have quickly been harnessed in the fight against the pandemic. Undoubtedly, this “third AI” boom will have a significant impact not only on healthcare but also on the completely new organization and quality of life in this “digitized to survive” world in which we currently live, and which we will discuss in the pages to follow.
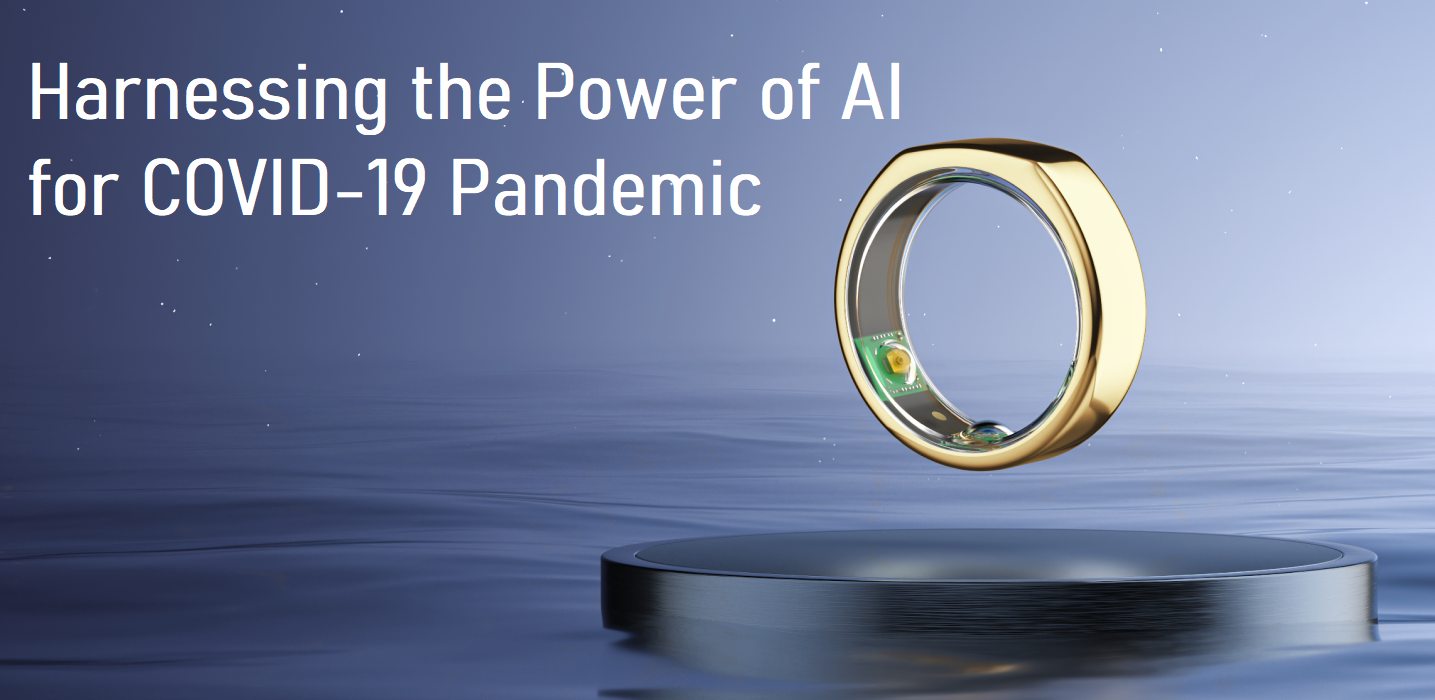
Although AI-empowered applications have been used experimentally during several disease outbreaks, the unprecedented spread of the COVID-19 pandemic mobilized all means to tackle different aspects of disease spread and treatment.
The rapid adoption and early results have demonstrated that AI could play an important role in the fight against COVID-19 and future disease outbreaks. Based on big data analytics, machine learning (ML) and deep learning (DL) methods and techniques, AI has proven helpful to identify the spread of the disease, its clustering, trends and patterns, as well as predicting future outbreaks and mortality rates, and support the diagnosis and monitoring of large numbers of cases, resources and supplies management and, of course, facilitating research for the prevention and effective treatment. Most of the applications evolved quickly from experimental to practical use and even proof-of-concept pilots turned to be of major help in the fight against the “unknown”.
AI for outbreak predictions
AI/ML was applied in various systems to forecast the spread of the virus, to produce early warnings and provide useful information about the disease outbreak and vulnerable regions, as well as for prediction of morbidity just by monitoring the social media platforms, news and posts.
The Canadian startup company BlueDot is credited with the early detection of the virus using an AI and its ability to continuously review over 100 data sets of news, airline ticket sales, demographics, climate data and animal populations. They spotted the outbreak of pneumonia in Wuhan, China on 31 December 2019 and identified the cities most likely to experience this outbreak.
Smart wearables for pre-symptomatic alerting
Coronavirus-infected people sometimes do not realize their symptoms for up to 5 days. In that situation, the virus can easily and asymptomatically spread to a larger circle of people. A smart wearable ring manufactured by the Finnish startup Oura, which records temperature, heart rate, respiratory rate and levels of activity, has been widely used for testing various AI-based algorithms for early COVID-19 infection alerting and reduce the spread of the virus. One of the models is claimed to predict the people infected within 24 h whether they have COVID-19 symptoms or not and detect fever before one has it. The Oura ring can continually register various rest-taken schedules, action-based types and their degree, the ecosystem temperature, and pulse fluctuation in the body. The data collected from 65,000 subjects as part of the TemPredict study will be stored at the San Diego Supercomputer Center and will be available to link with other datasets for further analyses. It is expected that AI/ML-based models analyzing the evolution and correlation of multiple parameters through data from wearable sensors, will help for the early detection of other infectious diseases, such as the flu.
Similar research at U.S. Army Medical Research and Development Command was piloted to monitor remotely the health status of the personnel and for AI-based early pre-symptomatic alerting. It is also expected that soon findings from this research will provide a near-continuous level of support and resilience to any U.S. Soldier across the globe.
Remote diagnosis and telemedicine
The widest spread of AI/ML empowered solutions for COVID-19 handling are the various telemedicine applications. By processing the data from remote sensors (like temperature, heart rate, respiration, and blood oxygen) such systems help clinicians to care for patients in their own homes, in nursing homes, in hospitals and to optimize the triage and the resource planning. Based on such parameters, but also on other remotely detectable health signs, such as voice, movement, weight, and even toileting, the ML models are used to monitor and predict the onset of adverse events and the progression of the disease. AI was used to augment and adapt mobile heath applications which use smart devices like watches, mobile phones, cameras and other wearables for diagnosis, efficient monitoring and contact tracing.
A non-traditional AI/ML-based method for early COVID-19 diagnosis was introduced by the MIT research team. It uses a novel approach for the early diagnosis of COVID-19 by analyzing the coughing as recorded and transmitted by a dedicated mobile application. The researchers have found that asymptomatic people differ from healthy individuals in the way they cough, although this is not decipherable to the human ear. The model is trained on tens of thousands of samples of coughs, as well as spoken words. It accurately identified 98.5 per cent of coughs from people who were confirmed to have COVID-19, including 100 per cent of coughs from people without symptoms however, tested positive. A cloud-based application is ready for production and further training on data sets (initially trained on more than 200,000 forced-cough audio samples). ML-based methods have been used in previous research to detect defects in human vocal cords by pronouncing different phrases, such as “mmm” or “them”, as well as for the early diagnosis of Alzheimer’s disease by analyzing emotional states in speech.
AI for monitoring cases and logistics
AI techniques are applied for monitoring patients in clinical settings and the prediction of the course of treatment. Based on the data derived from vital statistics and clinical parameters, AI was helpful in the decision-making for resource allocation and prioritization of the need for equipment, such as ventilators and respiratory supports in intensive care units. AI can also be used for predicting the chances of recovery or mortality in COVID-19 and to provide daily updates, storage and trend analysis, as well as for charting the course of treatment.
Researchers from Israel reported an AI model which predicts the length of COVID-19 hospitalization. They used AI/ML to track hospitalized COVID-19 patients between clinical states and predict the number of days expected in different states through a personalized model. The system was trained and validated through huge data sets from the Ministry of Health and the COVID-19 hospitalized patient registry, which includes patient age and sex in addition to daily clinical status and dates of admission and discharge.
Accelerating research for drugs and vaccines
Because of the unpredictable, yet highly-contagious nature of the COVID-19 virus, research for analyzing the structure of the virus to create drugs and effective vaccines became the highest priority.
The research is challenging since the virus belongs to a family of enveloped coronaviruses that contain single-strand RNA structures, and yet, similarly to double-stranded viruses, such as HIV, Ebola, and others, COVID-19 is capable of rapidly mutating, making vaccine development and virus analysis difficult. AI methods and tools are being used to support this research and accelerate vaccine development.
A successful implementation of the Linearfold algorithm, disclosed by Baidu to researchers, is significantly faster than traditional RNA folding algorithms at predicting a virus’s secondary RNA structure. Baidu AI scientists have used this algorithm to predict the secondary structure prediction for the COVID-19 RNA sequence, reducing overall analysis time from 55 minutes to 27 seconds, meaning it is 120 times faster.
The MIT researchers have used machine learning to identify medications that may be repurposed to fight COVID-19. They have developed appropriate cell culture models to validate the hypothesis for a correlation between viral infection/replication and tissue ageing and allow for highly specific and targeted drug discovery programs.
To help researchers generate potential new drug candidates for COVID-19, IBM has applied the novel AI generative frameworks to three COVID-19 targets and has generated 3000 novel molecules, shared to scientists. The researchers at the Quebec institute Mila have used ML to discover antiviral drugs to fight COVID-19, using graph neural networks to explore combinations of existing drugs and trying to search for all possible drug-like molecules.
AI/ML is helping in the race for the development of a vaccine against the pathogen. Researchers from the University of Michigan used their Vaxign reverse vaccinology-machine learning platform that relied on supervised classification models to predict possible vaccine candidates for COVID-19. Thus, AI has accelerated manifold the pace of discovery. The rapid development of two highly effective mRNA vaccines (from Moderna and Pfizer) was possible through AI technology and innovative collaboration among researchers around the world. Thanks to AlphaFold2, the AI system created by the London-based company DeepMind, it was possible to predict the three-dimensional structures of very challenging target proteins with high accuracy. It is also used to model the possible mutations of the virus and thus the improvement of the vaccines.
AI helps not only the discovery and evolution of vaccines. Moderna and IBM plan to use modern technologies, AI and blockchain, for smarter vaccination management, distribution, and supply chain management.
Chatbots and service robots
Chatbots have been quickly adapted for and widely used to disseminate information, especially in remote settings, as well as for symptom monitoring, behaviour change alerting, mental health support and remote assistance. However, researchers and authorities have warned about important challenges.
Providing reliable evidence-based information is critical in a pandemic but there could be issues, such as conflicting advice between global and local authorities, and misinformation. The developers should decide how to amplify the reliable sources and coordinate global information sources, such as the WHO, with advice from regional authorities.
Chatbots usually provide links to third-party services through which personal data might be shared with unexpected consequences. Symptoms’ screening and sharing health-related information between companies and governments are among the sensitive areas of application of these technologies. Besides, there is a boom of service robots and anthropomorphic robots with an AI core that can be used for the delivery of essential services and routine assistance.
The pandemic changed the AI and data analytics
The pandemic has changed the way AI was traditionally used for data analytics. Previous ML-based applications have been widely used for big data analytics, including Deep Learning techniques. According to Gartner, when COVID-19 hit, organizations using traditional analytics techniques that rely heavily on large amounts of historical data realized that many of these models are no longer relevant and a lot of historical data sets are useless. The forward-looking data and analytics teams are pivoting from traditional AI techniques relying on “big” data to analytics that requires less, or “small” and more varied data and apply adaptive machine learning. In addition to the expected technology scalability, responsible and ethical AI norms should be implemented to avoid data bias and provide data privacy.
AI against COVID-19 - Highlights:
- AI and control of COVID-19 article by the Council of Europe
- In Search for Cure: How Baidu is bringing AI to the fight against coronavirus
- AI for Computational predictions of protein structures associated with COVID-19
- IBM, Amazon, Google and Microsoft partner with White House to provide compute resources for COVID-19 research
- Predicting the evolution of the virus: The BlueDot case
- Using AI to verify the compliance with the anti-epidemic measures by phone
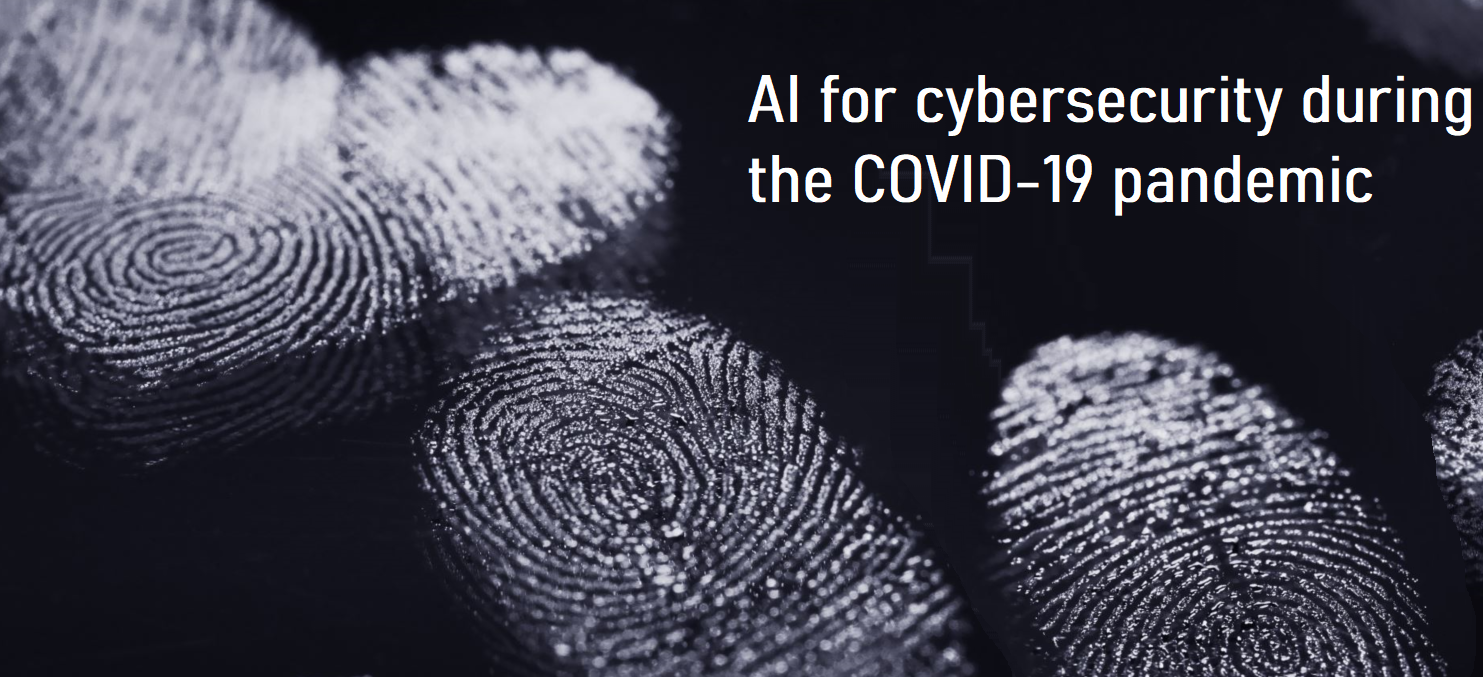
The year of the COVID-19 pandemic will certainly be remembered as the year in which cybersecurity events exploded and cyber incidents transformed the way we live and work. Due to the intensified use of the internet and virtualization, cyber incidents have also increased dramatically. More than 445 million cyberattacks have been reported in 2020, double in comparison with 2019. But not only the number and intensity increased, but also the scope and the sophistication of the attacks have noticeably evolved, the impact, as well as the motivation and the tools of malicious actors. Since the onset of the pandemic, the FBI has seen a fourfold increase in cybersecurity complaints and the global losses from cybercrime estimated at above $1 trillion in 2020. Several headlines already qualified 2020 as “The year that the COVID-19 crisis brought a cyber pandemic”.
Of highest interest were all types of knowledge, data and information, related to COVID-19 research, drugs and vaccines, test results and healthcare and patients’ records in particular. In July 2020, the UK National Cyber Security Centre (NCSC) reported that drug firms and research labs had been targeted for Covid-19 vaccine information by a group known as APT29 (Russian state-sponsored hackers).
AI/ML methods and tools are already widely used in incident detection and prevention systems (IPDS), as well as in more sophisticated and advanced SIEMs (Security Information and Event Management systems) for network and systems behaviour monitoring, filtering “false positives” and rapid response.
Due to the increased intensity of the attacks and the growing attack surface complexity, the AI/ML methods and tools became inevitable for threat assessment, effective cyber defence, threats assessment and resilience.
The main types of growing attacks in 2020 and some of the novel AI-based threat hunting methods and tools were:
- social engineering - a third of the breaches, of which 90% by phishing - AI is used to detect various AI-enabled attacks, like “deep fakes” (technology can determine when an image or video is counterfeit). AI/ML is used to filter out fake reviews in a dataset (e.g., statistics show 61% of electronics reviews on Amazon are fake), and misinformation.
- ransomware (just the ransom demands amounted to $1.4 billion, 22% of the cases) – in Germany, cybercriminals targeted a hospital for ransom, with patient care systems being disabled and resulting in one patient's death.
- DDoS attacks remain a growing threat, with 4.83 million DDoS attacks attempted in the first half of 2020 alone. Since criminals now employ AI to perform DDoS attacks, the AI/ML and behaviour monitoring tools are the cure to look for the weak spots, especially if there is a massive amount of data involved.
- third party software, supply chain and corporate security challenges – AI/ML/DL technology is for “hidden threats” analysis and remote working environment.
AI for Cybersecurity - Highlights:
- Sensitive Content Filtering with AI: Facebook is now using AI to sort content for quicker moderation
- Check Point Presents the First Autonomous Threat Prevention System
- Machine Learning: Higher Performance Analytics for Lower False Positives
- Applicability of machine learning in spam and phishing email filtering: review and approaches
- A Machine Learning Study on Phishing URL Detection
- Thorough Analysis For Using Data Science To Detect Malicious Domains
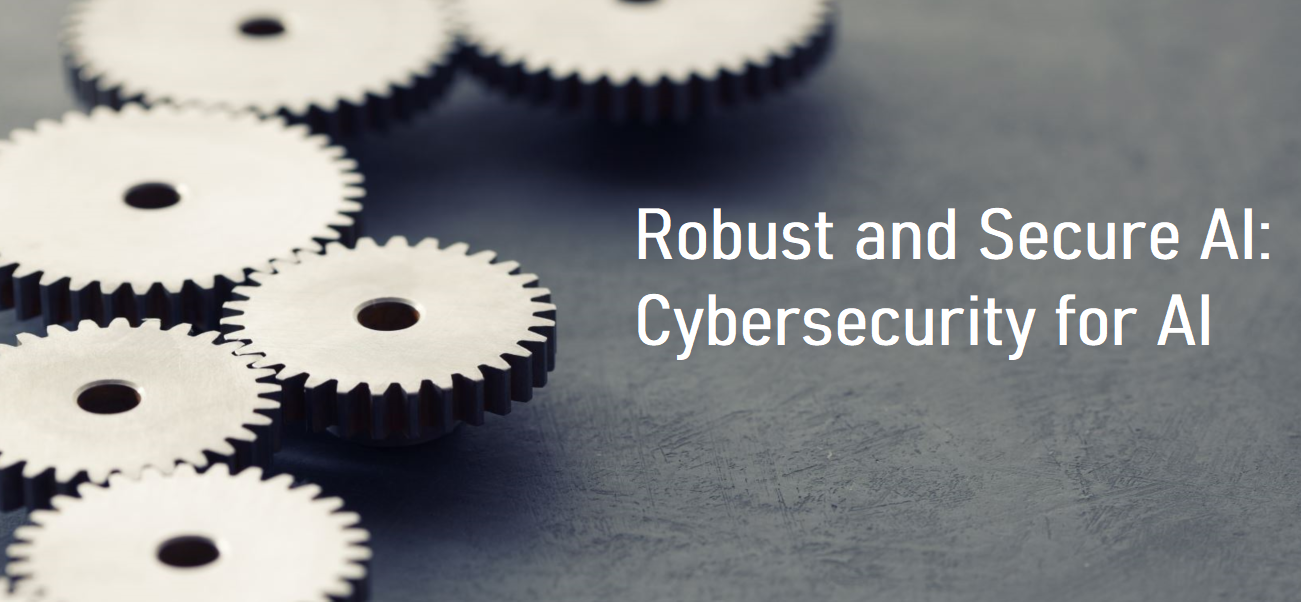
No doubt that AI systems being based on software and IT need to comply with evolving cybersecurity requirements. But this is not enough, as the AI methods and tools are based on a totally different from the traditional architectures, technologies, algorithms and data. The EU approach to AI, as outlined in the EU Strategy for AI from 2018, and in the EC White Paper of February 2020 on AI is defined as “ethical, secure and cutting-edge AI made in Europe”.
The Executive Director of the EU Agency for Cybersecurity ENISA Juhan Lepassaar said: “Cybersecurity is one of the bases of trustworthy solutions for Artificial Intelligence. A common understanding of AI cybersecurity threats will be key to Europe’s widespread deployment and acceptance of AI systems and applications.”
In the ENISA “AI Cybersecurity Challenges” report of December 2020, an AI cybersecurity ecosystem and a “Threat Landscape for AI” are outlined. It is stated, that “When considering security in the context of AI, one needs to be aware that AI techniques and systems making use of AI may lead to unexpected outcomes and may be tampered with to manipulate the expected outcomes. This is particularly the case when developing AI software that is often based on fully black-box models, or it may even be used with malicious intentions, e.g. AI as a means to augment cybercrime and facilitate attacks by malicious adversaries”. It is, therefore, essential to secure the AI itself.
The steps to achieve cybersecurity for AI, specifically tailored for machine learning-based models and the AI development and implementation lifecycle are:
- understand what needs to be secured (assets, subject to AI-specific threats and adversaries);
- understand the related data governance;
- manage threats in a multi-party ecosystem in a comprehensive way by using shared models and taxonomies;
- develop specific controls to ensure that AI itself is secure.
The cybersecurity threats to AI are listed as: “lack of robustness and the vulnerabilities of AI models and algorithms, e.g. adversarial model inference and manipulation, attacks against AI-powered cyber-physical systems, manipulation of data used in AI systems, exploitation of computing infrastructure used to power AI systems’ functionalities, data poisoning, environment variations which cause variations in the intrinsic nature of the data, credible and reliable training datasets, algorithmic validation/verification (including the integrity of the software supply chain), validation of training and performance evaluation processes, credible and reliable feature identification, data protection/privacy in the context of AI systems, etc.”
Cybersecurity is fundamental for trustworthy AI solutions, but three general aspects are listed in the “Ethics Guidelines for Trustworthy AI” by the EU High Level Expert Group as: lawful, ethical, and robust (technical robustness and safety, security and resilience, transparency, traceability, explain-ability, etc). Standards and certification schemes are under development by the standardization bodies (ETSI, CEN, ISO/ICE, others). An “Assessment List for Trustworthy Artificial Intelligence” (ALTAI) is available online for self-assessment, especially tailored for SMEs.
Cybersecurity for AI - Highlights:
- Microsoft announces two AI-based technologies to combat disinformation
- Attacking Artificial Intelligence: AI’s Security Vulnerability and What Policymakers Can Do About It
- Scalable Private Learning with PATE
- Employing Encryption Techniques on Machine Learning Training Data
- Early Warning System for Disinformation Developed with AI
- Scientists voice concerns, call for transparency and reproducibility in AI research
- Security software for autonomous vehicles
- Making AI Trustworthy
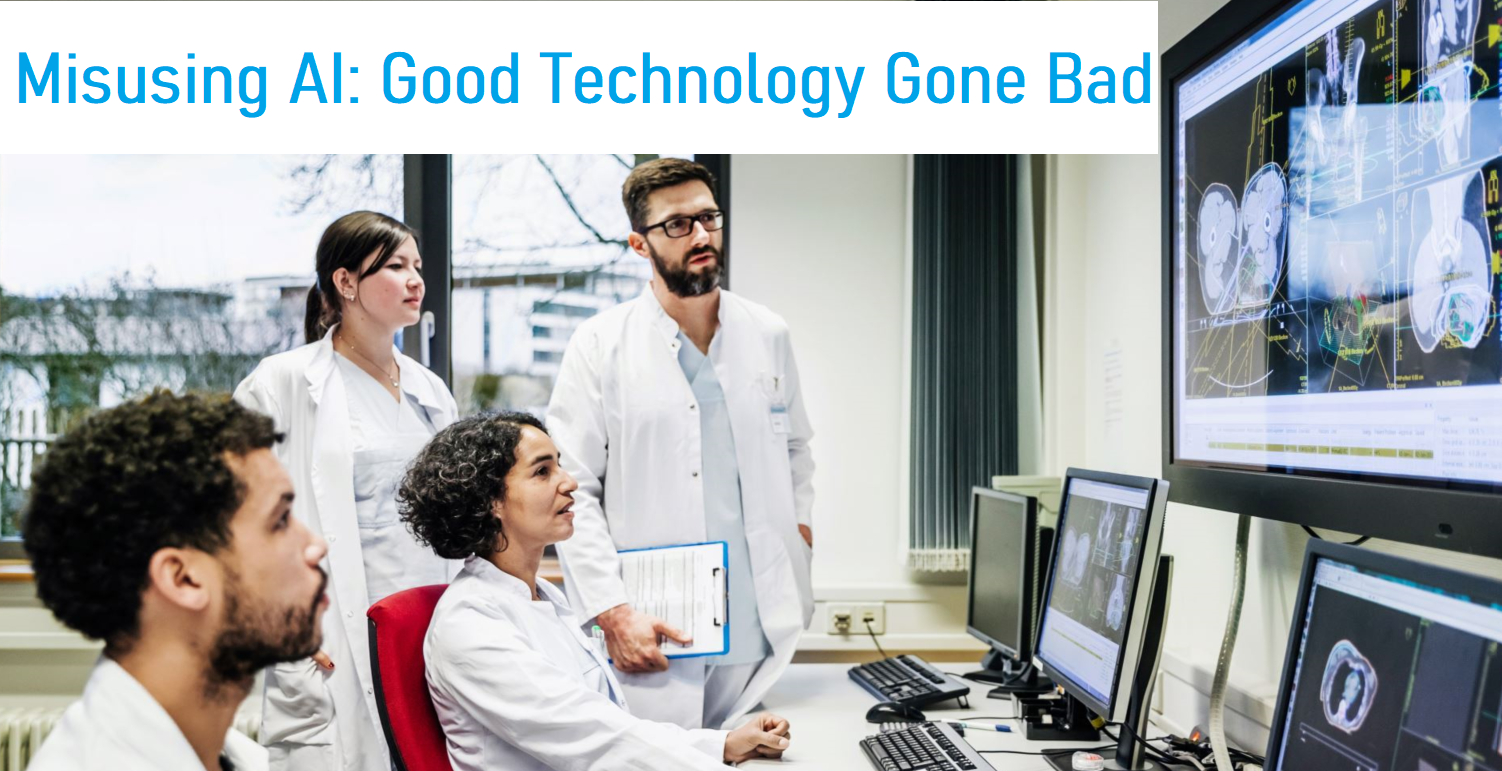
“AI will be either the best or the worst thing, ever to happen to humanity.” – Stephen Hawking
Now, more than ever, and especially in the light of a global pandemic, we are realizing the double-edged sword that AI is if misused. Privacy concerns, AI algorithms tracking our every move, have come to the media forefront and weaponizing AI has become increasingly scarier.
From using AI to remotely execute intelligent, self-propagating attack, to employing AI to track abidance to pandemic countermeasures, or using ML to mimic the behaviour of trusted system components, we have witnessed a lot of artificial intelligence misuse during 2020. And we are convinced now, more than ever, that AI will be either the best or the worst thing to ever happen to humanity.
Intelligent Surveillance
With recent developments in AI for video and audio analytics, the nature of what we think surveillance is, becomes subject to change. Experts worry that besides some positive outcomes to that, such as AI-powered cameras being able to recognize people breaking the law or posing an immediate danger to others, troubling predictions are also becoming a reality. With powerful algorithms being able to quickly identify people this data can be further correlated to other data about the same person, providing a very indiscrete insight into people’s lives, their motivation and behavioural patterns. Furthermore, with the increasingly cheaper and accessible cloud and hardware storage, video, audio and other artefacts of our every move are being stored for longer than they used to be, making it easier to “dig up dirt”, for instance.
Facial and Voice Recognition
With hundreds of bots, automatically scraping the web for video and audio recordings, along with images of people, an enormous amount of data is being processed and analyzed without people’s consent, creating vast facial or voice recognition databases for training a large variety of machine learning algorithms. This non-consensual collection of personal and sensitive data could put an end to privacy by falling into malicious hands or being used for questionable purposes. Besides, with the advancement of deep fakes, seeing no more equals believing and we become increasingly troubled when attempting to recognize fake news, footage, recordings and information.
Faking Medical Data and Images
Deep Learning malware samples have been tested specifically in medical environments, showcasing a variety of intelligent attacks against images, such as altering MRI scans, or even more scary, altering a patient’s diagnosis by recognizing and removing tumours from MRI scans. The possibility to seamlessly conduct intelligent AI-based attacks on entire systems-of-systems by intelligently mimicking components of a healthcare service or supply chain have also scared healthcare providers and cybersecurity experts alike.
Misusing AI - Highlights:
- Deep Learning Malware Can Fake Cancer on Medical Images
- Deepfakes Are Going To Wreak Havoc On Society. We Are Not Prepared
- Surveillance company harassed female employees using its own facial recognition technology
- AI Has Made Video Surveillance Automated and Terrifying
- Clearview AI stops facial recognition sales in Canada amid privacy investigation
- Protecting smart machines from smart attacks
- Security Attacks Analysis of Machine Learning Models
Latest in Cybersecurity
- How 30 Lines of Code Blew Up a 27-Ton Generator
- HAFNIUM targeting Exchange Servers with 0-day exploits
- Ransomware Hits Dozens of Hospitals in an Unprecedented Wave
- Over 2800 e-Shops Running Outdated Magento Software Have Been Hit
- North Korean Hackers Used 'Torisma' Spyware in Job Offers-based Attacks
- Two New Chrome 0-Days Under Active Attacks — Update Your Browser
Cybersecurity Institutions & Initiatives of the Issue: ESI CEE, CyResLab of ESI CEE, GCHQ and the Cybersecurity Lab at Sofia Tech Park
ESI CEE (European Software Institute — Center Eastern Europe), was established in 2003 as a public-private partnership initiative and excellence center by the European Software Institute, Spain (now Tecnalia), the Bulgarian Association of Software Companies (BASSCOM), Bulgarian ICT Agency, and ICT industry stakeholders.
Information security and resilience is a key strategic direction of ESI CEE’s research and development activities. In 2013 ESI CEE established the CyResLab (Cyber Resilience Lab) team in a strategic partnership with CERT at Carnegie Mellon University, Pittsburgh, USA.
ESI CEE has implemented cybersecurity audits and consultations together with private and public organizations in the following industry domains: finances, transport (incl. maritime), oil production, software development, and IT services, and education. Since 2015 ESI CEE manages the establishment and development of the Cybersecurity Laboratory at Sofia Tech Park, a project funded by the European Regional Development Fund and the Bulgarian state budget.
ESI CEE also has expertise in:
- Development of cybersecurity strategies and strategic thinking for IT businesses
- e-Governance
- Cybersecurity models, situation awareness, training, and education
- Process improvement models and standards
- IoT and robotics education
CyResLab (Cyber Resilience Lab) is the cybersecurity division of ESI CEE. The mission of CyResLab is to increase the competitiveness of digital enterprises and the resilience of digital ecosystems by making available various resources and services, designed to aid IT, software, and information security improvement. Since its establishment in 2013, the CyResLab team has developed a series of hands-on trainings and subsequent consultations that are regularly delivered to industrial and public clients. The trainings and consultancy services are specialized in the following areas:
- Resilience management and process maturity based on comprehensive and complete reference models to support the organizations in maintaining multi-standard compliance and resilience. Courses and consultations include risk management; process maturity (CMMI DEV and CMMI SVC); resilience management (RMM) and others.
- Web threats simulations (basic and advanced) including top threats and advanced attacks such as Network and crypto-threats, SQL injection, Broken session, and Authorization management, XSS, CSRF, Secure coding, and others.
- Mobile Security (separate editions for iOS/Android) including Insufficient Transport Layer Protection; Unintended Data Leakage; Broken Cryptography; Poor Authorization and Authentication and others.
- Networks Security: DDOS and methods for protection including DDOS and DDOS attack methods; Network/content service provider infrastructure; RTBH – Remotely triggered black hole; Self-adaptive systems and others.
The team of CyResLab has expertise and strong interests in various aspects of information security, such as cryptology, secure architectures, secure software development, threat modelling and others.
GCHQ (Government Communications Headquarters) is an intelligence and security organisation responsible for providing signals intelligence and information assurance to the government and armed forces of the United Kingdom. GCHQ was originally established after the First World War as the Government Code and Cypher School (GC&CS) and during the Second World War it was responsible for breaking the German Enigma codes.
Currently, there are are two main components of the GCHQ, namely 1) the Composite Signals Organisation (CSO), which is responsible for gathering information, and 2) the National Cyber Security Centre (NCSC), which is responsible for securing the UK's own communications. Among GCHQ’s core capabilities nowadays, is Artificial Intelligence, and more specifically:
- Fact-checking and detecting deepfake media;
- Mapping international networks that enable human, drugs and weapons trafficking;
- Analysing chat rooms for evidence of grooming to prevent child sexual abuse;
- How the National Cyber Security Centre could analyse activity at scale to identify malicious software to protect the UK from cyber-attacks.
In the beginning of 2021, GCHQ published a first-of-its kind paper outlining how they will use AI to protect the UK , laying out GCHQ’s AI and Data Ethics Framework, and how they intend to use AI in their operations.
In 2015, the Research and Development and Innovation Consortium of Sofia Tech Park and ESI CEE united their efforts to carry out joint activities and cooperate with the common goal to establish the Cybersecurity Laboratory as a leading research center in the field of cybersecurity. The laboratory currently works for the creation, development, and coordination of common national capacity in the following areas:
- Cyber-resilience and flexibility of information and management systems;
- Situational awareness of the levels and impact of the digital dependence of society and economy, and the consequent risks and cyber-vulnerabilities, their prevention, and the overall preparedness for cyber-attacks and incidents;
- Vulnerability research, threat modelling, and replication of cyber-physical systems with a potential cyber-hybrid impact;
- Development of standards and methodologies for the design, development, and protection of cyber-dependent critical systems and resources, digital ecosystems, and the overall improvement of the cybersecurity posture of IT-intensive systems.
The applied research of the Lab is focused on its potential uses for the development of modern training programs through simulations, practical classes, and exercises, as well as services in the field of cyber-resilience for the public and private sector.
MonSys is a web availability monitor, developed by the Cybersecurity Laboratory in the period 2019-2020, with financing from the Research and Development and Innovation Consortium of Sofia Tech Park and Nemetschek Bulgaria, with the support of the ESI CEE and CyResLab.
MonSys provides a flexible, robust, and scalable monitoring platform, as well as customizable alerting and situational awareness information and intelligence. The flexibility and scalability, being the core advantages of the platform is extremely effective in challenging areas, such as:
- Monitoring fleets of millions of IoT devices, in either push or pull mode;
- Collecting data on availability and/or security for entire vertical or horizontal supply chain segments;
- Extracting real-time data from highly specialized services that require specific test setup, process, or infrastructure.
With MonSys you can also perform custom availability checks for different types of infrastructure, such as various black-box, grey-box, and white-box availability checks/metrics.
Cybersecurity Institutions in Bulgaria and the European Union
- European Software Institute — Center Eastern Europe (ESI CEE)
- CyResLab of ESI CEE
- Cybersecurity Laboratory at Sofia Tech Park
- European Union Agency for Cybersecurity (ENISA)
- The ECHO Project (European Network of Cybersecurity centres and competence Hub for Innovation and Operations)
- EU High-Level Expert Group on Artificial Intelligence
- The European AI Alliance
- ETSI Technical Committee CYBER
- ETSI ISG “Securing AI”
- ENISA Working Group “AI Cybersecurity”
- CERT BG